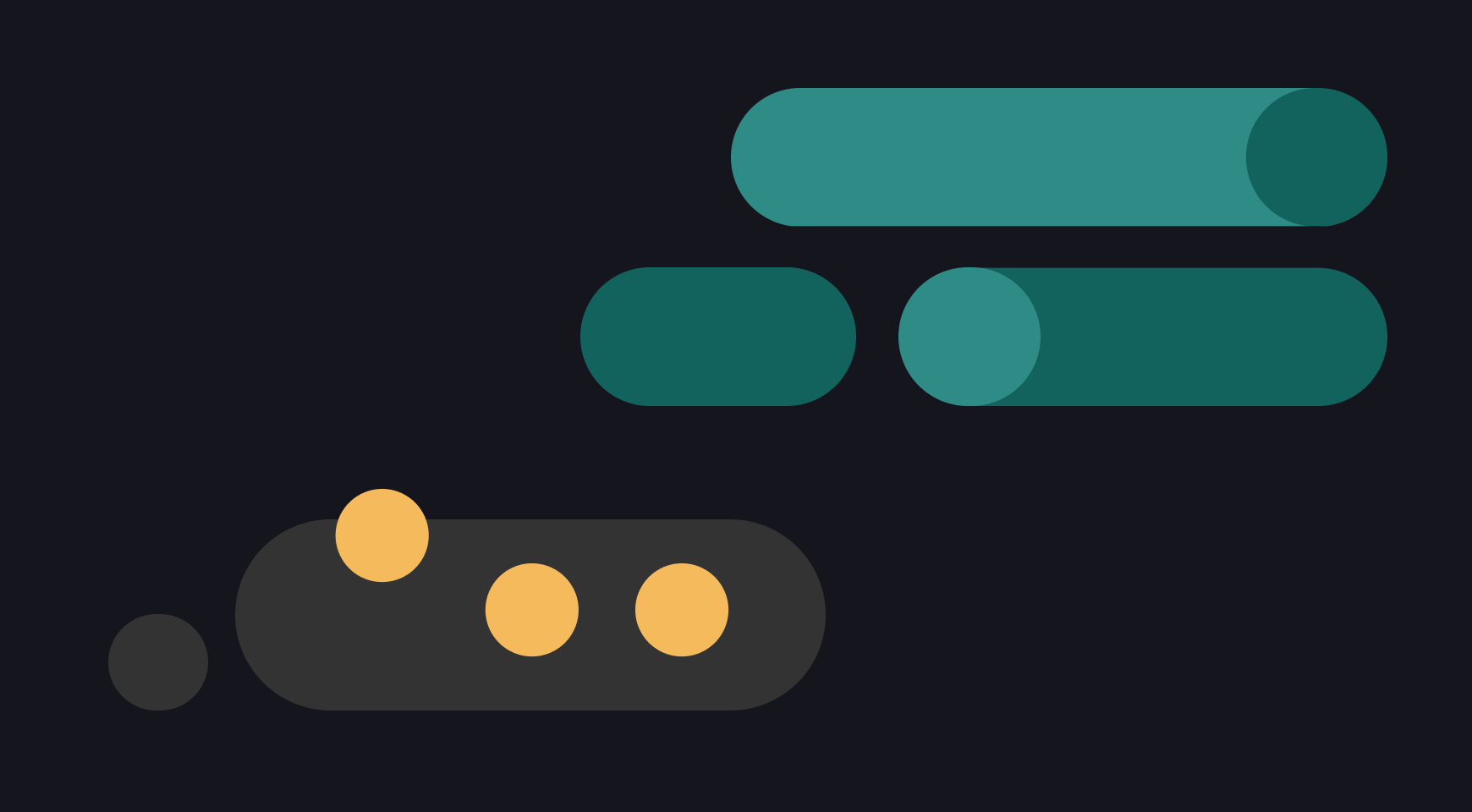
Open source LLM models have come a long way since their inception. Once confined to research labs, these AI systems have steadily grown in size and sophistication, now capable of processing vast amounts of information and generating human-quality text, translations, and even creative content.
LLMs underpin a wide range of applications: for example, here at Agente we used an LLM to build an AI chat assistant that automates a variety of tasks from summarizing research papers and translating texts to creating posts for social media and aiding in creative writing. The ability of LLMs to learn and adapt from massive datasets makes them valuable tools across various industries, from healthcare and education to finance and marketing.
This article delves into the world of the best open-source LLMs, highlighting the top 7 contenders for 2024. We'll explore the best large language models: breaking down their strengths and weaknesses, ideal user base, and potential use cases, helping you navigate this exciting and rapidly evolving field.
The Benefits of Open-Source LLMs
While both open-source and proprietary large language models exist, open-source models offer distinct advantages, making them an attractive option for many users. Here are some key benefits:
Transparency and trust
Open source LLM models allow users to inspect the underlying code and algorithms, fostering greater transparency and trust in their decision-making processes. This is particularly important for applications where ethical considerations and explainability are paramount.
Customization and control
The open source nature of these models empowers users to fine-tune them on their own datasets, tailoring them to specific needs and applications. This level of control allows users to address potential biases and enhance the model's performance for their unique use case.
Cost-effectiveness
Compared to proprietary models with associated licensing fees, open source large language models often require only investment in the necessary computational infrastructure, leading to potentially lower total cost of ownership. This makes them accessible to individuals, startups, and research institutions with limited budgets.
Community-driven development
Open-source LLMs benefit from the collective knowledge and expertise of a global developer community. This fosters continuous improvement through collaborative bug fixing, feature enhancements, and the creation of new applications and use cases for the models.
The List of Large Language Models
While there are numerous solutions on the market right now, those are some of the most prominent examples of open-source large language models, with each offering unique features and use cases.
Bard - (Google AI, released 2023)
Developed by Google AI, Bard stands out for its ability to access and process real-time information, allowing for responses that are grounded in current events and factual updates. This makes it a valuable tool for tasks requiring up-to-date information, such as summarizing news articles or generating content based on recent trends. However, Bard is still under development and may not be as comprehensive or polished as some other established models.
Jurassic-1 Jumbo (Microsoft, released 2022)
This Microsoft offering boasts an impressive size and diverse training data, allowing it to excel in tasks requiring factual language understanding and generation. Jurassic-1 Jumbo is well-suited for tasks like question answering and generating different kinds of creative text formats, such as poems or code. However, its large size can make it computationally expensive to run, limiting its accessibility for users with limited resources.
Megatron-Turing NLG (NVIDIA, released 2022)
Developed by NVIDIA, Megatron-Turing NLG focuses on generating different creative text formats, like poems, code, scripts, musical pieces, emails, and letters. Its strength lies in its ability to mimic various writing styles and generate human-quality creative text formats. However, it may not be as effective for tasks requiring factual accuracy or in-depth knowledge of specific domains.
WuDao 2.0 (BAAI, released 2021)
This BAAI-developed model is known for its multilingual capabilities, supporting over 100 languages. This makes WuDao 2.0 a valuable tool for tasks requiring translation or generating content in multiple languages. However, concerns have been raised regarding its potential for bias, as its training data might reflect biases present in the source material.
BLOOM (BigScience Research, released 2022)
Developed by a consortium of researchers, BLOOM is notable for its focus on responsible development and ethical considerations. It's trained on a carefully curated dataset designed to minimize bias and promote factual accuracy. This makes BLOOM an attractive option for users seeking transparency and ethical considerations in their LLM usage. However, its focus on ethical considerations may come at the expense of performance, since the developer uses quite restricted datasets to train generative AI model.
GPT-Neo (EleutherAI, released 2022)
Developed by EleutherAI, GPT-Neo is a large and versatile model well-suited for a wide range of tasks, including text generation, translation, and question answering. Its open-source nature allows for customization and fine-tuning, making it adaptable to specific needs. However, its large size can pose computational challenges, and its performance might not be as strong as some other models in certain specialized tasks.
T5-XXL (Google AI, released 2020)
This Google AI model is known for its ability to perform various tasks beyond just language generation. T5-XXL excels in tasks like question answering, summarization, and translation, making it a versatile tool for various applications. However, compared to some newer models, it might not be as strong in creative text generation or handling complex factual queries.
A Side-by-Side Comparison
To get a better idea of how different large language models stack up against each other, take a look at this over view table. It details each model’s advantages and disadvantages, along with who it’s intended for and best use cases.
LLM |
Pros |
Cons |
Target Audience |
Use Cases |
Bard |
Up-to-date information access, diverse tasks |
Under development |
Researchers, developers |
Summarizing news, generating content based on trends |
Jurassic-1 Jumbo |
Large size, diverse data, factual language |
Computationally expensive |
Researchers, enterprises |
Question answering, creative text formats |
Megatron-Turing NLG |
Creative text generation, diverse styles |
Limited factual accuracy |
Writers, content creators |
Poems, code, scripts, musical pieces |
WuDao 2.0 |
Multilingual capabilities |
Potential bias concerns |
Researchers, businesses |
Translation, multilingual content generation |
BLOOM |
Responsible development, ethical considerations |
Potentially lower performance |
Researchers, ethically conscious users |
Question answering, summarization, translation |
GPT-Neo |
Large, versatile, customizable |
Computationally demanding |
Developers, researchers |
Text generation, translation, question answering |
T5-XXL |
Versatile tasks beyond generation |
Less emphasis on creative text |
Researchers, developers |
Question answering, summarization, translation |
Trends and Innovations in LLMs
The LLM landscape is constantly evolving, with researchers and companies actively pushing the boundaries of these powerful models. Here are some key trends and innovations shaping the future of LLMs:
Focus on explainability and control
As LLMs become more complex, there's a growing emphasis on understanding their decision-making processes and ensuring they operate under human control. This involves developing techniques for explaining model outputs, identifying potential biases, and implementing safeguards to prevent misuse.
Multimodal LLMs
The integration of various modalities, like text, audio, and video, is gaining traction. These multimodal LLMs can process and generate information across different formats, enabling applications like generating captions from images or translating spoken languages.
Smaller and more efficient models
While large, powerful models continue to be developed, there's also a trend towards creating smaller, more efficient models. This makes LLMs more accessible for users with limited computational resources and opens up possibilities for real-time applications on mobile devices.
Collaborative development
Open-source communities are playing a pivotal role in LLM development. These communities foster collaboration, knowledge sharing, and rapid innovation, allowing researchers and developers to collectively build and improve models.
Responsible development and deployment
Ethical considerations are becoming increasingly crucial. Companies and researchers are actively implementing responsible AI practices throughout the LLM development process, focusing on mitigating bias, ensuring data privacy, and establishing responsible deployment guidelines.
Increased investment
Recognizing the potential of LLMs, major companies like Google, Microsoft, and NVIDIA are making significant investments in research and development. This influx of resources is accelerating the pace of innovation and pushing the boundaries of what LLMs can achieve.
These represent just a glimpse of the exciting developments shaping the future of LLMs. As the field continues to evolve, we can expect even more powerful, versatile, and responsible models that will revolutionize how we interact with technology and navigate the information landscape.
Challenges and Limitations of Open-Source Large Language Models
Despite their numerous advantages, open-source LLMs also face certain challenges and limitations that users should be aware of:
Computational requirements
Many open-source LLMs are large and complex, requiring significant computational resources to run effectively. This can be a barrier for individual users or organizations with limited computing power.
Technical expertise
Utilizing and fine-tuning open-source LLMs often requires technical expertise in areas like machine learning and data science. This can limit their accessibility for non-technical users seeking a user-friendly experience.
Security and bias concerns
As with any AI model, open-source LLMs are susceptible to potential security vulnerabilities and biases inherited from their training data. Mitigating these risks requires careful evaluation and ongoing vigilance by users and developers.
Limited support and maintenance
Compared to proprietary models with dedicated support teams, open-source LLMs may have limited ongoing support and maintenance resources. This can pose challenges for users encountering technical difficulties or seeking assistance with specific use cases.
Ethical considerations
While some open-source projects, like BLOOM, prioritize ethical development, others might not have the same level of scrutiny. Users need to be responsible for assessing the potential ethical implications of using any open-source LLM, including potential biases or alignment with the project's values.
By acknowledging these challenges and limitations, users can make informed decisions about whether open-source LLMs are suitable for their specific needs and resources. It's crucial to carefully evaluate the trade-offs between the benefits of open-source models and the potential challenges they present.
The Bottom Line
As the field of LLMs continues to grow and evolve, open-source models are poised to play a significant role in democratizing access to this powerful technology and fostering innovation across various domains. By understanding the benefits, limitations, and current landscape of open-source LLMs, users can make informed decisions about whether these models are suitable for their specific needs and contribute to the responsible development and deployment of this transformative technology.
If you’re looking to get more out of open source LLM models — feel free to reach out! We provide comprehensive consulting on AI models, as well as build custom solutions based on existing offerings.
Rate this post!
672 ratings, average ratings is 5.0 out of 5
Frequently asked questions
Whether you represent a private business, a large enterprise or an educational institution, our e-learning platform development services will greatly improve the performance of your company.
Is there a free LLM model?
Yes, there are several free, open-source LLM models available. However, it's important to understand that "free" in this context doesn't necessarily mean there are no associated costs. While you won't pay a licensing fee, you might need to invest in computational resources and technical expertise to run and fine-tune the model respectively.
Which LLM is the best?
Determining the "best" model depends entirely on your specific needs. Each has unique strengths, like creative text generation or factual tasks. Consider factors like computational needs, technical expertise needed, and potential biases when choosing the LLM that best aligns with your specific requirements and priorities.
Related Posts
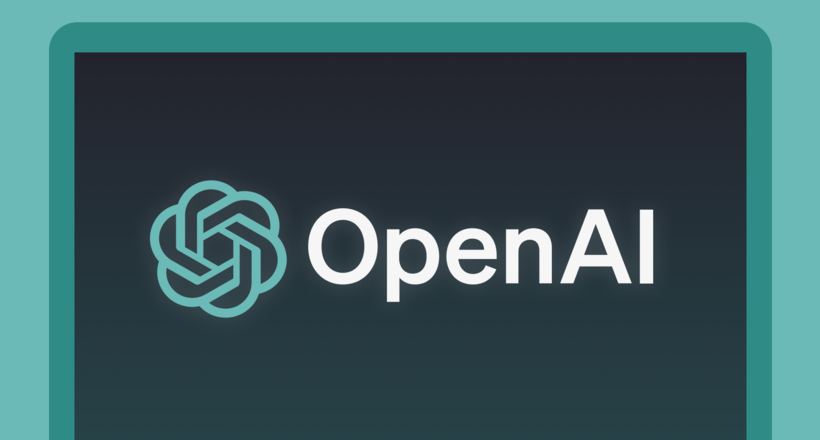
16 January 2024
ChatGPT Plugin Development: Features and Benefits for Business
Explore the process of crafting a ChatGPT plugin tailored precisely to meet your unique business requirements.
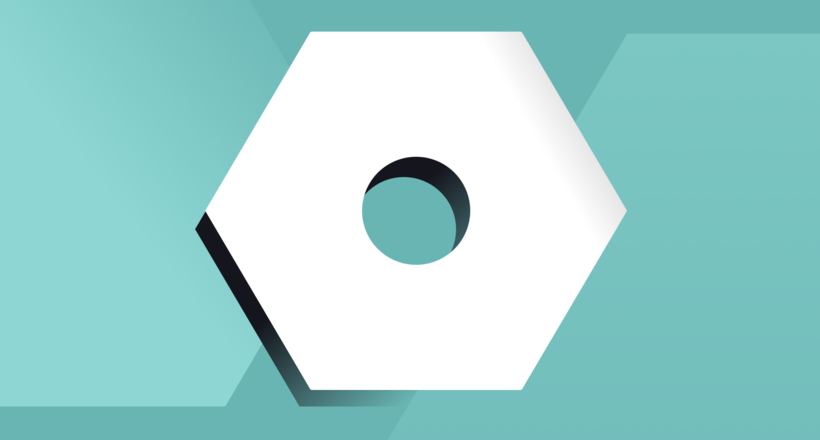
19 January 2024
AI Model Fine-Tuning: How to Use Your Organization's Data?
Organizations are transitioning from generic solutions to hyper-customized intelligence, seeking AI models designed to address their unique challenges and propel them toward strategic objectives.
This demand has propelled fine-tuning to the forefront of AI development.
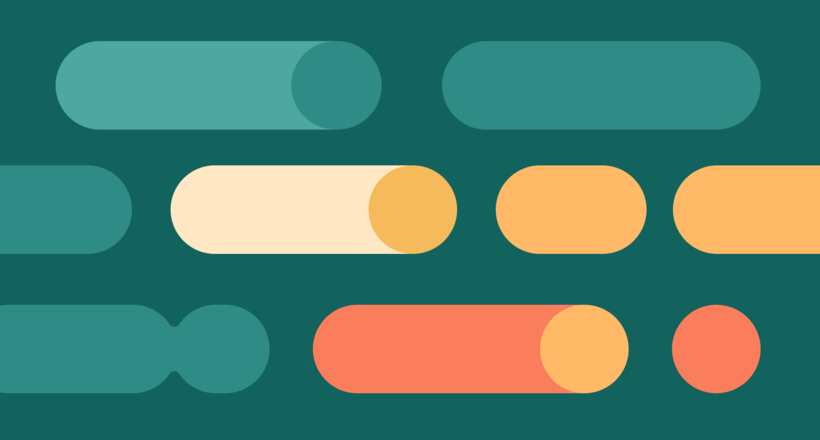
07 May 2024
What are large language models: a complete guide
Get your large language model definition straight: in this article, we cover the concept of LLMs, their capabilities, types, and challenges.
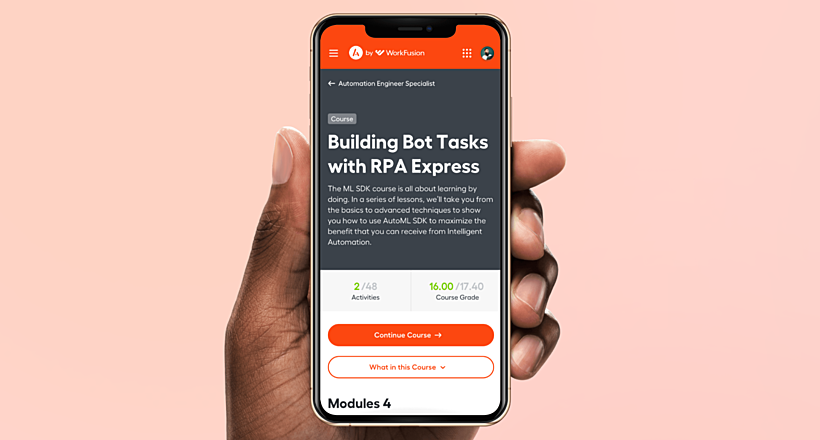
Develop Custom Corporate Microlearning Platform
Custom microlearning solutions for corporate training: Discover how to develop a tailored platform for efficient and engaging employee learning
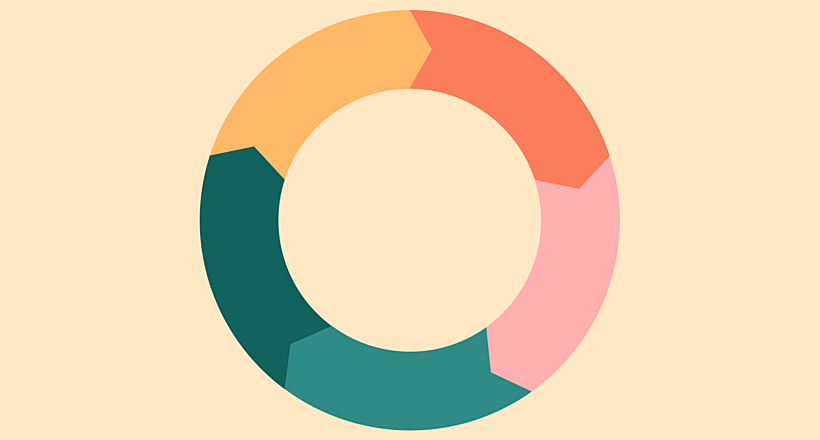
24 January 2024
Employee Training Management Software Development in 2024: Features and Cost
Streamline your employee training with cutting-edge software solutions. Explore the features and costs of employee training management software.
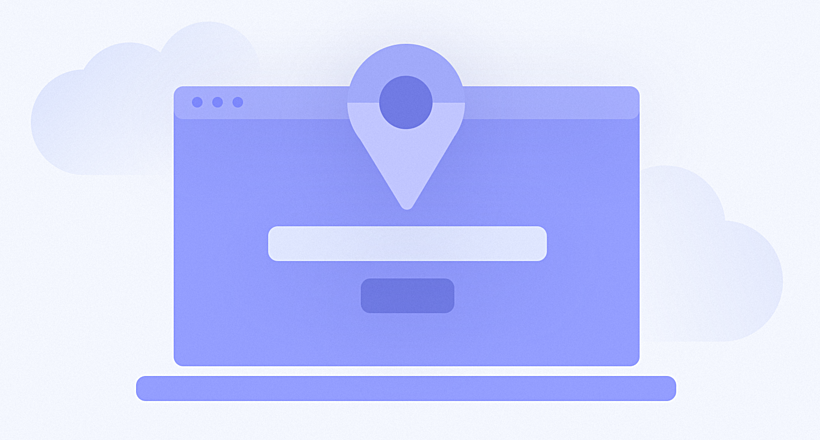
How to Launch Your Online Travel Marketplace Platform in 2024?
Learn how to launch your own online travel marketplace platform in 2023 with our comprehensive guide and step-by-step insights.
Let's talk
Is there a challenge your organization or company needs help solving? We’d love to discuss it.
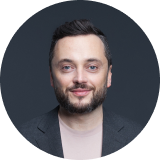
Managing Director, Partner
Andrew Terehin
Thank You!
Your message has been successfully sent.
We will contact you very soon.